Quantile Regression Explained in 5 Minutes
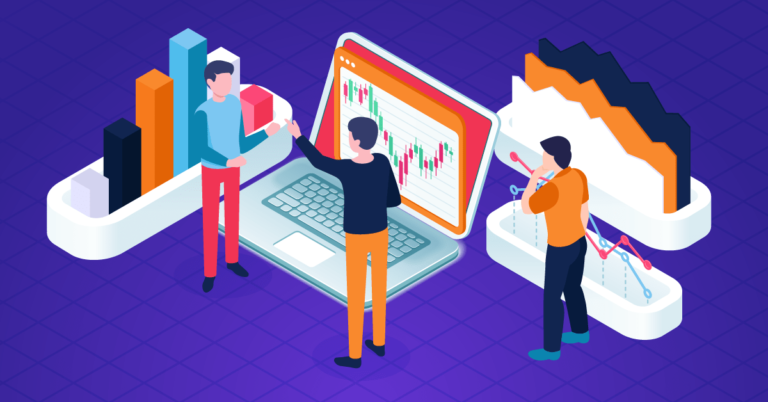
Linear regression has its loopholes in analyzing data across a specific distribution. One of them is having a fixed mean across the whole data. However, quantile regression has advantages over linear regression. It explores different medians across different data distributions, and each median is known as the conditional median.
This article explores different questions that will help you get started with quantile regression, its applications, when to use quantile regression, and a few essential questions.
What is Quantile Regression?
Understanding the meaning of quantile regression is critical to thoroughly grasping the detailed information about it. As a result, it is crucial to define quantile regression as it is an excellent alternative to using linear regression in data analysis. But first, let us define quantile and regression.
Quantile is the point in a data distribution corresponding to data values in distribution divided equally in a corresponding order. A percentile is a type of quantile that divides a variable into 100 equal places.
For every given sample, the middle value of such data is called the median, middle quantile, or 50th percentile.
Regression is a statistical measure used for establishing a pattern quantitative analysis showing the tendency of a data variable to return to a median range. It is the process of using data to predict the likelihood of future events to occur around a median value. These variables leading to this prediction can be dependent or independent.
Now, what is quantile regression?
Quantile regression is the regression technique employed when linear regression could not satisfy its assumptions. Quantile regression determines the median of a set of data across a distribution based on the variables within that distribution. It is an extension of the linear method of regression.
This explains why the averages of quantile analysis are not constant as against the linear regression method.
A quantile regression example is the case of a selling price prediction for houses in the real estate market.
Questions arise to challenge how accurate your predictions can be. You may not trust your guts, but you can prove your predictions to be an exact answer with quantile analysis.
After calculation in each area of the market, you could reach different means, considering the conditions of the market. These means of a quantile regression are what is known as “conditional means.”
What is Quantile Regression Used For?
No company across industries in the corporate world will thrive more than those leveraging their data to predict possible scenarios in the sectors and how they can tackle them. Even small or local businesses leverage data analysis to improve business profits through business intelligence analysis. Although it may not exactly be like quantile regression interpretation, their analysis is vital for business success.
We will consider a few applications of quantile regression across various industries. That is because the importance of quantile regression requires more emphasis.
Quantile regression application in finance and economics
Financial evaluations are put in place by banks after critically examining their “value at risk” models. These risk measures reduce or eliminate the financial industry’s market risk. Importantly, these deduced risk models are from the quantile analysis conducted through a regression approach. As a result, banks are aware of the variables affecting the data distribution in a particular quantile.
Quantile regression is used to determine market volatility and observe the return distribution over multiple periods.
For the economic application, quantile regression influences different variables on the consumer markets. In this regard, individuals are grouped into three different categories; low-income, medium-income, or high-income groups.
As such, quantile regression is an essential analytical tool for determining income distributions of the labor economics population. This can give more insights into the kinds of tax policies or social policies that their implementation will result in a sustainable economic situation.
Quantile regression application in environmental modeling
Hydrologists are constantly concerned about creating models for rainfall and river flow. Their concerns center around the need to design reservoirs that will help alleviate water scarcity during droughts and design drains that will help channel water appropriately during high rainfalls. This requires statistical assessment by a data analyst to provide detailed possibilities while leveraging the quantile regression approach.
Quantile regression application in medicine
Applying quantile regression in medicine has helped public health professionals to determine the ignored variable resulting in a high value of a condition across a data distribution. This is applicable in medical diagnosis where certain variables are present in one tail of the distribution.
An example includes determining a disease condition while vigorously examining a dependent covariate such as age, including conditional distribution.
Quantile regression application in survival analysis
If you have ever experienced an event where the survival chance of an individual is a debate like the following day’s newspaper headline, you would agree that having exclusive access to data will give you more leverage to make an accurate survival analysis.
This involves examining the impacts of a particular covariate in determining an individual’s survival time. This may have different effects on individuals based on their levels of exposure to risks. As such, you may study the functions of different quantiles on the survival time at low-, medium- and high-risk conditions.
Quantile regression application in education
The last application of quantile regression to discuss is its application in education. Quantile regression is applicable in determining the factors responsible for students’ success or failure rates in a particular exam. This may depend on a response variable in some cases. Nonetheless, it involves extracting your data from a conditional distribution.
An example is of two students who performed well in an exam. Let’s say student A has a 1950 score, and student B has a 1680 score. We would say student A performed excellently and better than student B. But when we consider their ages, there may be questions regarding their individual conditional quantile spaces.
We will compare the score of student A with students of his age and compare the score of student B with students of his age. As a result, we are aware of the variable causing such difference in the data distribution.
What Does Quantile Regression Tell?
In contrast to the linear regression approach which employs least squares to determine the conditional mean of a specific distribution while considering different features as variables, quantile regression determines the median of a particular distribution from a much larger dataset. This median is known as the conditional median.
When to Use Quantile Regression?
One vital thing to understand is the allowance quantile regression presents you in understanding the functions of a non-conforming variable occurring across a data distribution. Some variables may not behave normally and have an efficient linear relationship with predictable variables. Quantile regression makes you understand their impact.
As a result, below are some reasons that may exist for you to use quantile regression.
1. When the linear regression assumptions are meeting up with each other.
2. When you have an outlier in your data sample.
3. To determine the median of a quantile within a data set.
4. When the error variables make your outcome variable spike up.
5. When you have abnormal residuals.
How Do You Interpret Quantile Regression in SPSS?
Interpreting quantile regression output in SPSS depends on understanding the relationships between assumptions and outliers (or influential cases) in your dataset. You must study the output by evaluating your assumptions while you identify possible outliers and influential cases whether you are interpreting standardized regression coefficients or unstandardized partial regression coefficients.
These regression coefficients are variables selected based on your assumptions that they are continuous variables. Likewise, you consider their influence on the output before giving a definite answer to the changes across a distribution.
What is Multivariate Quantile Regression?
Multivariate quantile regression is a regression approach that allows you to explore different probabilities and analytical methods within a quantile as it establishes critical connections across various depths. Likewise, you can refer to multivariate quantile regression as multiple output quantile regression.
Is Quantile Same as Percentile?
If you are worried about the difference between quantile and percentile, we will define each for your understanding. However, be aware that both are of the same meaning and interpretation but expressed as different terms.
Quantile is a distribution across a data sample where the entire distribution is divided into several equal parts. A percentile is the percentage value of that distribution. It is obtained by dividing the distribution in 100 places.
What is Quantile Loss?
First, another name for quantile loss is the quantile regression loss function. A quantile regression loss function predicts different quantiles while minimizing or maximizing measurable functions across the distribution. Importantly, quantile regression loss function helps to establish trust where linear regression could not.
Wrap up
The quantile regression approach has various advantages over linear regression compared to those mentioned in this article. Likewise, its applications across industries have established the importance of quantile regression. However, there is little to acquire from this single blog post.
Check out our learning paths to start taking courses in Data Science, business intelligence, deep learning, artificial intelligence, and other certification.
Subscribe to our newsletter to receive up-to-date information about advancing your Data Science career.
Also, don’t forget to share this article with your network of Data Science enthusiasts.