“Shades of Blue” Experiment and What it Means to a Data Scientist
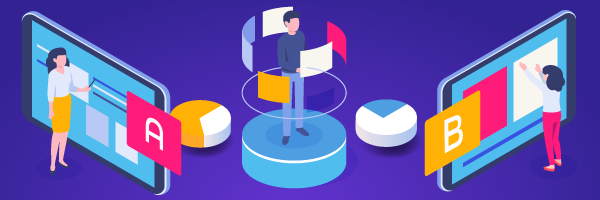
The difference between blue and green is clear and it’s pretty much a straightforward decision when deciding whether a blue or green tie matches your shirt. But what if deciding on an exact shade of blue is the difference on whether a company earns an extra $200 million profit?
Can such minuscule details such as a slight variation in color, border size or text really turn metrics around? Google Shades of Blue experiment is evidence that it can. The company was able to isolate a particular shade of blue that appealed to the most users and attracted more clicks. They used this color appeal to encourage users to click on ads, increasing their revenue significantly.
But most importantly, the experiment serves as irrefutable evidence of the power of data. Data analysts can learn a thing or two from the Google Shades of Blue experiment and that is what we explore in this article.
The 41 Shades of Blue Experiment
In 2009, a team at Google led by Marissa Meyer who was head of product at that time, carried out an experiment to determine the right shade of blue for ad links. The idea received much criticism for its statistical approach from designers who believed it was a waste of time and resources.
The company’s decision to agree with its engineers and allow the experiment caused a lot of in-house tension. Doug Bowman, who was the chief designer, left the company that year. He mentioned that the experiment contributed to his decision. According to him, debating on whether a border should be 3, 4 or 5 pixels wide was tiring and there were “more exciting design problems in this world to tackle.”
In 2014 Dan Cobley, former managing director of Google UK, spoke about the company’s choice of putting data above the supposed expert opinion of the highest-paid person on the design team. He explained that before the experiment the company put up ads on Gmail, just like they already had on its search page. They discovered that when they put the similarly designed ads from Gmail and search side by side, the blue text links were actually different shades.
The head designer could have simply chosen one shade and applied it to the other so the difference could be eliminated, the company chose to make a data-backed decision instead. Here’s what Google did, they allowed 1% of users to see the blue originally from Gmail on Google search as well and another 1% had the blue from Google search now showing in Gmail as well.
To be sure that they were using the right shade of blue of all shades available, Google carried out 40 more experiments, using every shade of blue imaginable. The experiment has come to be known as the “41 shades of blue experiment”.
Google’s data experts analyzed the click metrics for each shade tested and found that a purplish shade of blue has the most clicks. It had beaten a greener shade of blue to be the most click-inducing shade. The company switched the text link colors in Gmail and Google search to the winning shade and bagged an extra $200m a year in revenue.
A/B Data Science Testing
The Google blue color experiment is a form of testing known as A/B testing. It basically involves presenting users with two options of the same element or function and picking the most effective one according to metrics. Companies that are wondering how to get more clicks apart from ad relevance and website structure, may want to borrow that page from Google’s book.
A/B also known as split testing is a widely used method employed in user experience research. This test is mostly used to verify the acceptability of new products or features, especially in a user interface, marketing, and eCommerce.
Almost any user experience research problem can be put through A/B testing, from screen size, web page design, a form or text. The important thing is that the two items being tested are variants of the same variable.
These variations, classified as A and B, are presented to two portions of users. The first group or control group portion is directed to variable A and the other experimental group to variable B.
A/B testing is simple and can be applied in almost every business or organization that would benefit from data-backed decisions.
Types of A/B Testing
Below are some types of A/B tests. Each type is best applied to a specific situation. Knowing which to apply in what situation can help to avoid pitfalls and wrong results.
The Classic A/B test
This type of A/B test presents users with two variations of your web page at the same URL. With the classic test, you can compare two variations of the many different elements at the same time since a web page contains a large number of elements.
The Redirect test
If more than one new page is being launched at the same time on one server, the redirect test is recommended. The redirect test takes users to one or several distinct URLs.
Multivariate test (MVT)
Multivariate type of A/B testing works for checking user reactions to changes on a web page. With MVT, a web page can change its text color, banner style, or arrangement and measure the metrics from each change.
What exactly can be A/B tested?
A/B tests can be used to evaluate visible and invisible changes. Adding new UI design elements or changes in design layout, color changes or text are visible changes. Invisible changes can be new recommendation algorithms or page loading time.
Google’s 2009 experiment with the color blue is a good example of visible change while Amazon’s experiment with page load time is an example of invisible change. Amazon’s A/B test was a 100-millisecond increase in page load time, the results showed the reduced speed brought down sales by 1%.
What can’t we test?
Say you put up an entirely new UI system or completely replace an element for all users at once, you can not really use an A/B test to draw any comparative conclusion between the old and the new. This is because you would be testing a new experience without testing the old one in real-time. Metrics can be affected by any number of things, like a new market trend, public health issues, social unrest and if the results for A and B are not gotten concurrently, then the results are not valid as A/B test-derived.
Additionally, a new experience can bring about total aversion where users don’t like changes and since there is no old design to go back to they completely withdraw from using the service. Also, trigger a novelty effect where users are simply clicking just to test out everything, this will trigger short-lived metric spikes.
Choosing the Metrics
When setting the standards for metrics that would be relevant to A/B tests, sensitivity and robustness need to be considered. Sensitivity refers to the capacity of the selected metrics to capture the affected changes and robustness means that metrics shouldn’t completely ignore irrelevant effects.
To ensure that sensitivity and robustness are considered in metric selection, filtering and segmentation can be applied as the control and experiment are being created. User age, gender, device type, internet browser, device type are some factors that can be used to filter and segment.
What steps are involved in A/B testing?
Hypothesis
The hypothesis is the beginning of every experiment. Here, the null hypothesis and alternative hypothesis are stated.
A/B testing challenges the null hypothesis that observed differences between the two groups are purely random and proves whether the difference can be associated with a particular change.
Experiment design
At this stage, variants A and B are strategically placed so that they are available to two separate user groups. Also, the method and criteria for collecting data are determined here.
Data collection
Once the experiment has been set up, data collection begins. Data collection is often the toughest part of the data scientist’s job.
Inferences
After data has been collected, the data scientist gets to work drawing conclusions and making inferences based on the data analyzed.
Data-driven Decision Making
Data-driven decisions add value to a company’s services, it gives better direction for digital marketing efforts and contributes to revenue development. For some businesses, reliable data could mean the difference between failure and success.
Businesses depend on data scientists to help them navigate the world of big data. Data scientists have to gather, analyze and restructure copious amounts of data so that they can provide organizations with actionable plans and evidence-based predictions.
Google is well known for its commitment to data-driven decisions and if the tech giant could go the long way to avoid the HiPPO effect in color choices, smaller businesses ought to learn from them.
Conclusion
Of course, not every company can make millions of dollars in revenue simply by applying A/B testing like Google did but data-based decisions can help to increase overall company performance and provide an edge over competitors.
For data scientists, this demand for data analysis skills means that they have to stay on top of things in the world of data. Key players!
You too can be a key player in the Data Science field. Join the SDS Club to have access to hundreds of courses and be a part of a growing community of data scientists.
Subscribe to our newsletter to get real time updates on Data Science industry trends and best practices.
Also, share this post with your friends on social media. Let the good go round!