The Whys and the Hows of Nonparametric Statistics
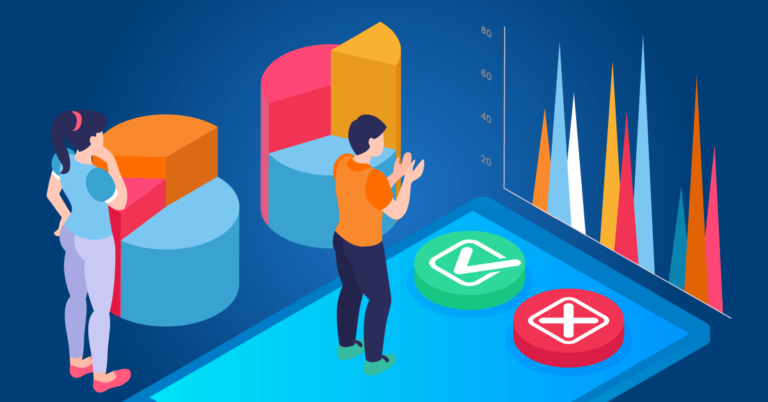
As a data scientist, understanding nonparametric statistics is an imperative. With the growing demand for data scientists, analysts, and data-focused engineers, you can’t afford to be at a disadvantage by neglecting the need to know this type of statistics.
In this article, you will learn the hows and whys of nonparametric statistics as well as the difference from its parametric counterpart.
So, to begin…
What are nonparametric statistics?
Nonparametric statistical (NPS) methods describe methods where assumptions about underlying statistical data distributions are not made. This method of statistics relies more on ranking than numbers. Therefore, it is best for data distribution that follows a natural order.
The difference between parametric and nonparametric statistics
The primary difference between parametric and nonparametric statistics, parametric methods of statistical analysis involve the assumption of data distributions. These assumptions made about observed data are used to estimate the parameters of the data under study.
Parametric statistics deeply depend on the values of data distribution. As such, it focuses on measures of value distribution like the average of data distribution, each variable’s variance from the mean, the general standard of deviation from the mean, and all other measures of value distribution.
Nonparametric analysis, on the other hand, focuses on the ranking or order of data distribution and makes no assumptions of such distribution. To do this, statisticians take into consideration every value of the dataset and analyze the fluidity (order) of the distribution. Non parametric statistical analysis reveals the pattern of distribution. It shows the curves and shapes of a distribution in a chart or graph instead of estimating its parameters. Some examples below will better explain how this is possible. Further along in this article, you will understand why this type of statistical analysis is important to data scientists.
Types of nonparametric statistics
There are two types of nonparametric models of statistical analysis. The first method seeks to discover the unknown distribution pattern of the underlying data while the second is more focused on making statistical inferences about the underlying data regardless of their nominal values.
In the case of discovering the distribution pattern of the underlying data, statisticians often use histograms and Kernel methods to estimate the values of the parameters. Arguably, this falls short of the nonparametric model. However, when a second model is used to infer the ranking or order of data variables with little to no consideration of the parametric values, as seen in skewness, all of nonparametric statistics rules are followed.
So, if NPS methods care less about the nominal value of data distribution and only its ranking, why then are they so crucial in a data scientist’s knowledge arsenal?
Why use nonparametric statistics instead?
One of the major reasons nonparametric analysis is considered a better measure of data distribution is because it presents a complete dataset in comparison with its median value. Unlike its parametric counterpart which estimates the mean of a distribution and measures the variance of each data variable from that estimated mean, the NPS method of analysis consolidates the general dataset to help discover the ranking of variables as against their quantitative values.
A dataset of annual salaries, for example, often has a right-skewness. Most of the values cluster around the median salary. Using nonparametric statistics to analyze the earning pattern of a population gives a clearer better picture. However, because a few members of every population earn outrageously higher than the median salary, you will often notice a rapid spike in value in a salary distribution plot. Parametrically analyzing the distribution, in this case, can provide an estimate (mean, variance, or standard deviation) that shows little about the population’s earning pattern but instead generalizes its wealth level.
A typical case study is observed in the annual salary of San Francisco residents. Parametrically, San Francisco residents earn $101,000 on average, according to Payscale. However, a majority of the city’s population earns below that average. Yahoo Finance reported $74,841 as the median salary.
Although parametric statistics are regarded as a more reliable statistical measure because it brings all values into consideration, NPS goes beyond the quantitative analysis of the dataset by eschewing assumptions to reveal pain points in a distribution. By using nonparametric statistical methods, the dataset reveals that over half of the city’s population earns below the average.
Where are nonparametric statistics used?
NPS has several use cases. In these situations, it appears to be more instrumental in analyzing a dataset than parametric methods.
1. Data distribution with extreme values
For distributions with extreme values, as explained in the San Francisco salary distribution, the mean is not a reliable measure of central tendency as the dataset is significantly skewed rightward. When a situation like this occurs, parametric assumptions are usually unsatisfied. Therefore, nonparametric statistical methods are more trustworthy measures to use.
2. A tiny population size
Analyzing a dataset collected from a large population size can favor assumptions since each variable carries a substantial frequency. However, in a very small-sized population, parametric assumptions may be misleading and a widely inaccurate method of statistical analysis.
3. Nominal and ordinal data
When working with a distribution of continuous data, it is satisfying to make assumptions, and as such parametric methods could be applicable. Nominal and ordinal data, on the other hand, don’t work well with assumptions. Therefore, analyzing a dataset of ordinal and nominal data requires nonparametric statistical methods of analysis.
Nonparametric statistics examples
There are several nonparametric statistics examples. Each of these examples has varying use cases. However, they are very useful for analyzing datasets where parametric methods are inappropriate.
Mann-Whitney U Test
This is similar to the independent samples t-test. The only difference, in this case, is that this Mann-Whitney U test analyzes the relationship between two independent samples with ordinal data that cannot be accurately analyzed with a parametric method.
Wilcoxon Signed-Rank Test
This is a paired difference test used to compare two samples of repeated measurement, related or closely matched. This method is employed to assess the disparity between the means of these samples and reveal the order of this difference along with the data distributions.
The Kruskal-Wallis Test
This test is used to analyze both continuous and ordinal data. It is used to determine the differences between two or more groups of independent variables in a dataset. It is an extension of the Mann-Whitney U test but only applies to independent ranks in a single direction. Thus, it is often regarded as the nonparametric alternative to the one-way ANOVA test.
Mood’s Median Test
This is a rather simple nonparametric statistical model used to compare the median of two independent variables to assess their difference and ranking in a larger data distribution.
Friedman Test
Developed by Milton Friedman, this nonparametric model is an alternative to the one-way ANOVA for repeated measures. This test is used to assess the difference between groups of data with ordinal variables. It’s also applicable to continuous data in special cases. When continuous data spur assumptions that are unsatisfactory for running a one-way ANOVA on repeated variables, this Friedman test is often employed as a more accurate alternative.
The Sign Test
This nonparametric statistics method operates on a hypothesis that a null outcome is possible when the difference between compared medians is zero. It is an alternative to a simple t-test or the paired t-test. This Sign test is used to compare the sizes of two groups of nominal or ordinal data variables by comparing the difference in their median distributions. However, it can also be used to analyze a categorical dataset.
Conclusion
Nonparametric statistics are an essential analytic skill for every data scientist. Building a career in data science will expose you to tons of datasets, most of which will require you to employ multiple statistical methods to analyze. Being well grounded on non parametric statistical analysis will put you at an advantage when fazed by a set of variables with unreliable parametric assumptions, data distributed among a tiny population, or ordinal/nominal data.
Like all other proficiencies, nonetheless, mastering NPS requires training and commitment. SDSClub provides resources and courses created by data science experts to help get ahead in your career as a data scientist. Various expertly curated resources on all of nonparametric statistics are available for your use.
You can easily stay up to date on our posts by subscribing to our newsletter. Also, don’t forget to share this interesting resource with your friends. Share now!