When AI Meets BI: The Rise of Augmented Analytics
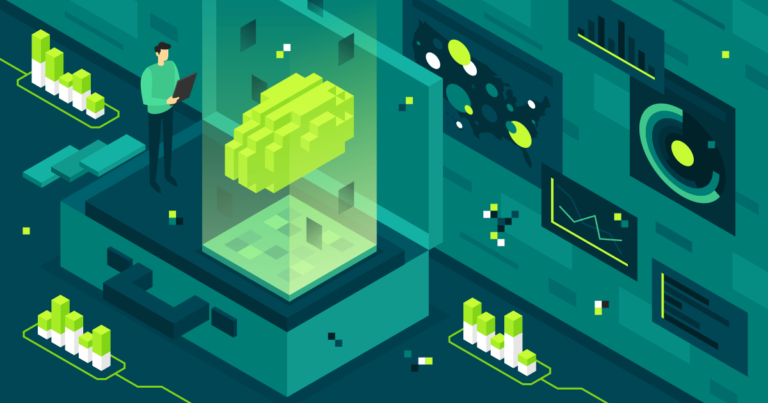
Do you need more insights from your business data? You’ve seen how many businesses use Business Intelligence (BI) and it’s hard to ignore the buzz around Artificial Intelligence (AI). But what happens when you marry these two disciplines? You get augmented analytics.
Augmented analytics is fast becoming an essential part of many business’s arsenals to gain valuable insights into their business. Combining existing technologies in AI with BI, a mainstay of getting insightful information from business data, augmented analytics promises an exciting future for businesses wanting to be more data-driven.
But what exactly is augmented analytics? How does it work and how do we use it? In this article’s we’ll delve into the details surrounding what could be the future of data analysis.
What Is Augmented Analytics?
A formal definition for augmented analytics is the use of technologies such as machine learning and AI to assist with data preparation, insight generation, and insight explanation to augment how we explore and analyze data in BI platforms and how we gain insights from it.
That is a mouthful, but what does it all mean? What does augmented mean? A plain definition of augmenting is to make greater, more numerous, larger, or more intense. In augmented analytics, it means that you improve data analytics with the use of machine learning tools and AI. Let’s explore this a little further.
We all know data is useless to a business if it cannot extract meaningful insights from it. Traditionally businesses could extract insights from data by employing data scientists or staff to interpret the data. For many businesses, the benefits of data remain locked because of the inability to gain insights from the data. This can be because of an inability to appoint data scientists or a lack of experience or knowledge.
Augmented analytics attempts to solve this problem by allowing businesses to extract insights from data using machine learning tools and AI.
How Do You Use Augmented Analytics?
Everyone agrees that data analytics is essential for any business. It has the potential to increase revenue, decrease expenditure, and, sometimes, turn around a struggling business. Through data analytics, a business can see how it’s performing, its marketing effectiveness, and how it compares to its competitors. The problem is that many businesses struggle to gain insights from their data. As mentioned, data, without insights is useless to a business.
Say, for instance, you run a business and your data reveals that your revenue is down compared to previous years. On face value and with no other data, it might seem that your business is struggling. Digging deeper you realize that, overall, the market has taken a downturn and is slower than other years. This new data shows that your business is not struggling. Without this insight, you could have panicked.
Conversely, your data shows that the market is as strong as ever. This means you’re making fewer sales because your marketing is not that effective or that your products are no longer in demand. Under these circumstances, you can act by increasing your marketing efforts or sourcing new products. This data is thus actionable.
This is a simple example, and you can imagine that in real-world situations you’ll often deal with much more data from many other sources. The process of analysis and extracting insights will also be far more complex. This process, after you’ve sourced the data, usually involves:
- Cleaning the data to prepare it for analysis
- Conducting the analysis
- Generating the insights, and
- Communicating the insights to all the role players for them to take action thereon.
To follow this process and gain insights from the data, you need a data scientist. Apart from being rare, they are also expensive. For many businesses, it’s thus not possible to appoint a data scientist. Even if a business could use a data scientist, many don’t have the business knowledge to interpret the data. They would thus need to work closely with executives to take the insights from the data and convert it into a business plan.
Augmented analytics solves this problem by reducing a business’s reliance on data scientists. It automates the process mentioned above, enabling the generation of insights automatically through the use of advanced machine learning and artificial intelligence algorithms.
Real-World Use Cases
Now you know what augmented analytics is and how it’s used. In real-world applications, it falls into 5 major categories. Let’s look at some of these real-world use cases.
1. Data preparation
By using augmented analytics, you reduce the time analysts take to clean and prepare the data. In general, analysts take up to 80% of their time with this task. By automating this process and using augmented data discovery, you free up analysts to spend more time gaining insights from the data.
2. Improve data interaction
Augmented analytics makes it easier for users to interact with the data. This makes it also possible for users with little experience in data analysis to extract insights from the data. The user simply has to speak or type a question to get a meaningful result from the query.
3. Enabling citizen data scientists
With the improved interaction with data and decreased need for experienced users, augmented analytics broadens the user base of analytics products. This allows companies to hire candidates who don’t have experience in statistics and mathematics. Less technical users can thus query the data and offer insights and recommendations based on the data.
4. Keeping tribal knowledge
By using augmented analytics, companies can guard against the loss of tribal knowledge because of a big part of the experienced workforce retiring.
5. Automation of insights
Machine learning tools can automate certain types of analysis that would take a data science team months to build on their own. This can prove valuable and automate insights based on data allowing executives to confirm suspicions around certain intuitions on the fly.
Benefits of Augmented Analytics
From what you’ve read, you’ll already have an idea of the benefits and possibilities that augmented analytics offers. Some of the pertinent ways in which it can benefit a business is:
- Deeper data analysis. Because the process is automated, a business can work through more data quicker. This augmented data discovery allows exhaustive data combinations and data comparisons which allow a business to know exactly which factors are influencing its business and in what way.
- Faster results. As we’ve seen, data analysts no longer have to spend their time cleaning and preparing data. The insights are delivered quicker and enable users to get answers to their questions on the fly.
- Better use of resources. Because data analysts are not bogged down with data cleaning, preparation, and questions, they can spend their time focussing on research that machines can’t yet do.
- Actionable insights. It simplifies the data analytics process. You get powerful insights into the business quicker. It thus enables you to make crucial business decisions quicker.
- Increase data literacy. It enables all users, whether experienced or not to gain value from data.
Keep in mind that these benefits are not an exhaustive list and that many other benefits will arise as the technology develops. What is certain, though, is that the existing benefits can form the basis of a successful business strategy.
Challenges And Best Practices
By now you might think that augmented analytics is the perfect solution to a business’s data analytics needs. There are, however, some challenges that might prevent a business from adopting it in their business. Certain best practices may, to some extent, overcome these challenges.
In the first place, a business needs its data to be accurate and error-free. If not, this could lead to inaccurate insights. Inaccurate data may also lead to data bias where the context from the surrounding data is missing. One way to counter this is to start small and move on to larger projects as success is achieved on the smaller projects.
In this respect, a business should also maintain its data to make sure the training data is up to date. If the training data is not up to date, the insights obtained from it won’t be worth much.
Another stumbling block a business may encounter is the equipment needed to perform the analysis. Analyzing huge amounts of data requires substantial processing power and the amount of data affects the response time to queries. Under these circumstances, starting small may also be advised.
Users should also be able to extract relevant results from the data. A business should therefore test its data to ensure it delivers relevant results. If it doesn’t, users will stop using it. Here it may be helpful to encourage a data-driven culture, promoting the use of analytics by all users in the organization.
What The Future Holds
Although augmented analytics is this relatively new, it is exciting to imagine what the future holds. With its benefits for business, it promises to bring about a revolution in data analytics.
As the technologies surrounding it develops, more and more benefits will become apparent. At this stage, it’s cheaper, easier, and faster than existing methods of data analytics and many businesses will use it for years to come. It is the future of data analytics.
Conclusion
You can see how augmented analytics has the potential to change the data analysis landscape. It’s also one of many technologies that make it an exciting time for machine learning and artificial intelligence practitioners and enthusiasts.
If you’re interested in machine learning, artificial intelligence, or business intelligence and you want to learn more, why not subscribe to SDSClub newsletter. There is a lot of cool information.
Leave a comment